Artificial Intelligence and Machine Learning in the Early Stage of a Product Life Cycle
Artificial Intelligence and Machine Learning
The interest in using data to create knowledge is not a novelty. With the accumulation of uncountable amounts of data, the challenge becomes how to capitalize it and evaluate the right data. This is called Machine learning (ML). ML originated in 1943 when neuroscientists Walter Pitts and Warren McCulloch tried to map humans’ thinking and decision-making processes using a neural network (1). The concept of a machine that is “learning” came with the findings of Alan Turning seven years later (2). Since then, it has become a motivator in exploring artificial intelligence (AI). AI and ML are concepts that are very often combined and confused. In a nutshell, machine learning is considered a subset of AI; it is the process of using mathematical models to help a computer learn and improve continuously without direct instruction based on its experience. Models that involve a composition of learned functions or concepts need a deep learning approach, a type of machine learning and an approach to AI. Specifically, it is a technique that represents the world as a nested hierarchy of concepts, with each concept defined concerning simpler concepts and more abstract representations computed in less abstract ones (3).
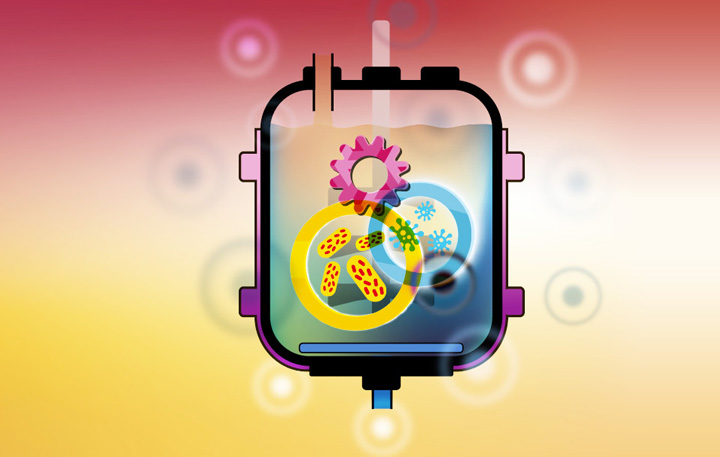
In pharma and biopharma, almost 70% of all manufacturing data collected is not used or not good enough to use (4). To optimize their processes, many manufacturers see the advantages of using the available data to gain insight into processes and operations. The ability to use this data, however, is not straightforward. Each system has its proprietary format, and a single unit operation can have several parallel systems generating data simultaneously. The solution proposed by Industry 4.0 to overcome the challenges of proper data collection and aggregation is by using big data and the Internet of Things (4). The shift to Pharma 4.0 requires a different look over manufacturing and process data capture. The use of AI to transform process data into knowledge that supports critical activities like process optimization and continuous improvement, at the same time, is compliant with data integrity guidelines (5), whichis a necessary step in the future of the industry (4). In this article, we will focus our analysis on the application of AI and ML in the early stage of the product lifecycle.
Applications in Early Drug Screening
The first or early stage of a product lifecycle is the development stage. Several works have shown the application and usage of AI and ML for early drug screening. The first example is AlphaFold, an algorithm developed by DeepMind that is able to accurately predict the 3D structure of a protein based on its amino acid sequence (6).
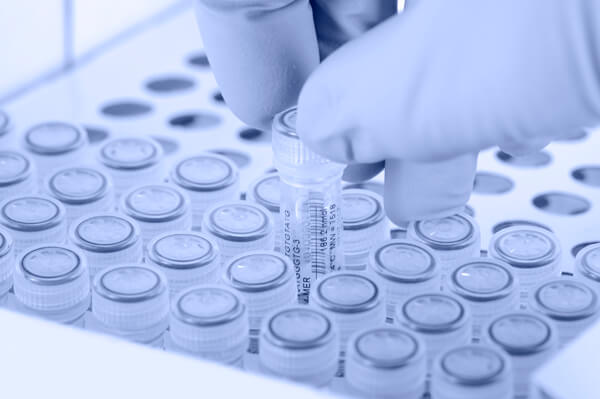
Another application is the use of predicting a drug’s pharmacometrics (application of models for disease or pharmacological measurement) (7,8,9). These tools are also helpful for performing target discovery (the choice of novel biomolecular drug targets), compound screening or finding associations between protein and phenotypes or functions (9,10). Several works apply AI and ML to quantify a compound druglikeness and developability. Druglikeness is the likelihood of a novel molecule becoming a drug based on multivariate similarity with other drugs. AI can guide the selection of the molecules to synthesize to obtain desirable drugs, using the molecular properties of each drug (10,11,12). As for developability, it refers to the compound’s feasibility to advance through the early discovery to development based on physicochemical and manufacturability properties such as yield, bioactivity and aggregation propensity (10,13). A concise review, including timelines of the use of generative adversarial networks for drug discovery, is provided By Zhavoronkov et al., (10). He and other authors were the first to develop a drug active in vivo based on AI (14).
Due to the ability of AI and ML to make sense of thousands of predictors and determine the relationship between them and the outcome, they can also be used to find the association between a drug and the response, to predict drug toxicity based on quantitative structure-activity relationships and predict biomarkers and clinical endpoints (7,8). This ability to streamline big data analysis renders these tools invaluable when working with omics tools (genomics, transcriptomics, proteomics, metabolomics, etc.) or expression data (9,10).
Finally, using AI tools can help predict clinical trial outcomes, potentially saving millions of dollars in clinical trial failure due to inefficient patient selection, recruiting and the inability to properly monitor patients (10).
Nonetheless, the disadvantage of using AI for in vivo and in vitro testing is the time needed to confirm the model predictions since it is necessary to produce the molecule before the testing. Besides, information on why a drug failed a clinical trial is often unavailable due to proprietary reasons, leading to a lack of data for building new models.
Want to know More?
Are you interested in knowing more about AI and ML? At ValGenesis, we are at the vanguard of implementing ML in the pharma and biopharma landscape with our team of highly experienced consulting experts. If you want to discover how ValGenesis Consulting successfully collaborated with a customer to implement a comprehensive developability scoring system using machine learning, our colleague, Daniel Pais, will present it at the 2023 PDA BioManufacturing Conference, which takes place in Seville, Spain, September 12-13.
References
- McCulloch, W. S., & Pitts, W. (1943). A logical calculus of the ideas immanent in nervous activity. The Bulletin of Mathematical Biophysics, 5(4), 115–133. https://doi.org/10.1007/BF02478259.
- Frankenfield, J. (2022, August 13). The Turing Test: What Is It, What Can Pass It, and Limitations. https://www.investopedia.com/terms/t/turing-test.asp#toc-the-bottom-line.
- Goodfellow, I., Bengio, Y., & Courville, A. (2016). Deep Learning. MIT Press. http://www.deeplearningbook.org.
- Manzano, T., & Langer, G. (2018). Getting ready for pharma 4.0. Data integrity in cloud and big data applications. Pharmaceutical Engineering, 72–70. https://www.ispe.gr.jp/ISPE/02_katsudou/pdf/201812_en.pdf.
- U.S. FDA. (2016). Data integrity and compliance with CGMP Guidance for industry. https://www.fda.gov/files/drugs/published/Data-Integrity-and-Compliance-With-Current-Good-Manufacturing-Practice-Guidance-for-Industry.pdf.
- Jumper, J., Evans, R., Pritzel, A., Green, T., Figurnov, M., Ronneberger, O., Tunyasuvunakool, K., Bates, R., Žídek, A., Potapenko, A., Bridgland, A., Meyer, C., Kohl, S. A. A., Ballard, A. J., Cowie, A., Romera-Paredes, B., Nikolov, S., Jain, R., Adler, J., … Hassabis, D. (2021). Highly accurate protein structure prediction with AlphaFold. Nature, 596(7873), 583–589. https://doi.org/10.1038/s41586-021-03819-2.
- Liu, Q., Zhu, H., Liu, C., Jean, D., Huang, S., ElZarrad, M. K., Blumenthal, G., & Wang, Y. (2020). Application of Machine Learning in Drug Development and Regulation: Current Status and Future Potential. Clinical Pharmacology & Therapeutics, 107(4), 726–729. https://doi.org/10.1002/cpt.1771.
- Liu, Q., Huang, R., Hsieh, J., Zhu, H., Tiwari, M., Liu, G., Jean, D., ElZarrad, M. K., Fakhouri, T., Berman, S., Dunn, B., Diamond, M. C., & Huang, S. (2023). Landscape Analysis of the Application of Artificial Intelligence and Machine Learning in Regulatory Submissions for Drug Development From 2016 to 2021. Clinical Pharmacology & Therapeutics, 113(4), 771–774. https://doi.org/10.1002/cpt.2668.
- U.S. FDA. (2023). Using Artificial Intelligence & Machine Learning in the development of drug and biological products - Discussion paper and request for feedback. https://www.fda.gov/media/167973/download?attachment.
- Zhavoronkov, A., Vanhaelen, Q., & Oprea, T. I. (2020). Will Artificial Intelligence for Drug Discovery Impact Clinical Pharmacology? Clinical Pharmacology & Therapeutics, 107(4), 780–785. https://doi.org/10.1002/cpt.1795
- Kadurin, A., Aliper, A., Kazennov, A., Mamoshina, P., Vanhaelen, Q., Khrabrov, K., & Zhavoronkov, A. (2017). The cornucopia of meaningful leads: Applying deep adversarial autoencoders for new molecule development in oncology. Oncotarget, 8(7), 10883–10890. https://doi.org/10.18632/oncotarget.14073
- Muehlemann, N. (2023). ML-Based population selection and enrichment in drug development. Biopharmaceutical Report, 30(I), 16–22.
- Bailly, M., Mieczkowski, C., Juan, V., Metwally, E., Tomazela, D., Baker, J., Uchida, M., Kofman, E., Raoufi, F., Motlagh, S., Yu, Y., Park, J., Raghava, S., Welsh, J., Rauscher, M., Raghunathan, G., Hsieh, M., Chen, Y.-L., Nguyen, H. T., … Fayadat-Dilman, L. (2020). Predicting Antibody Developability Profiles Through Early Stage Discovery Screening. MAbs, 12(1). https://doi.org/10.1080/19420862.2020.1743053
- Zhavoronkov, A., Ivanenkov, Y. A., Aliper, A., Veselov, M. S., Aladinskiy, V. A., Aladinskaya, A. V., Terentiev, V. A., Polykovskiy, D. A., Kuznetsov, M. D., Asadulaev, A., Volkov, Y., Zholus, A., Shayakhmetov, R. R., Zhebrak, A., Minaeva, L. I., Zagribelnyy, B. A., Lee, L. H., Soll, R., Madge, D., … Aspuru-Guzik, A. (2019). Deep learning enables rapid identification of potent DDR1 kinase inhibitors. Nature Biotechnology, 37(9), 1038–1040. https://doi.org/10.1038/s41587-019-0224-x