Getting Closer to AI Adoption in the Pharmaceutical Industry
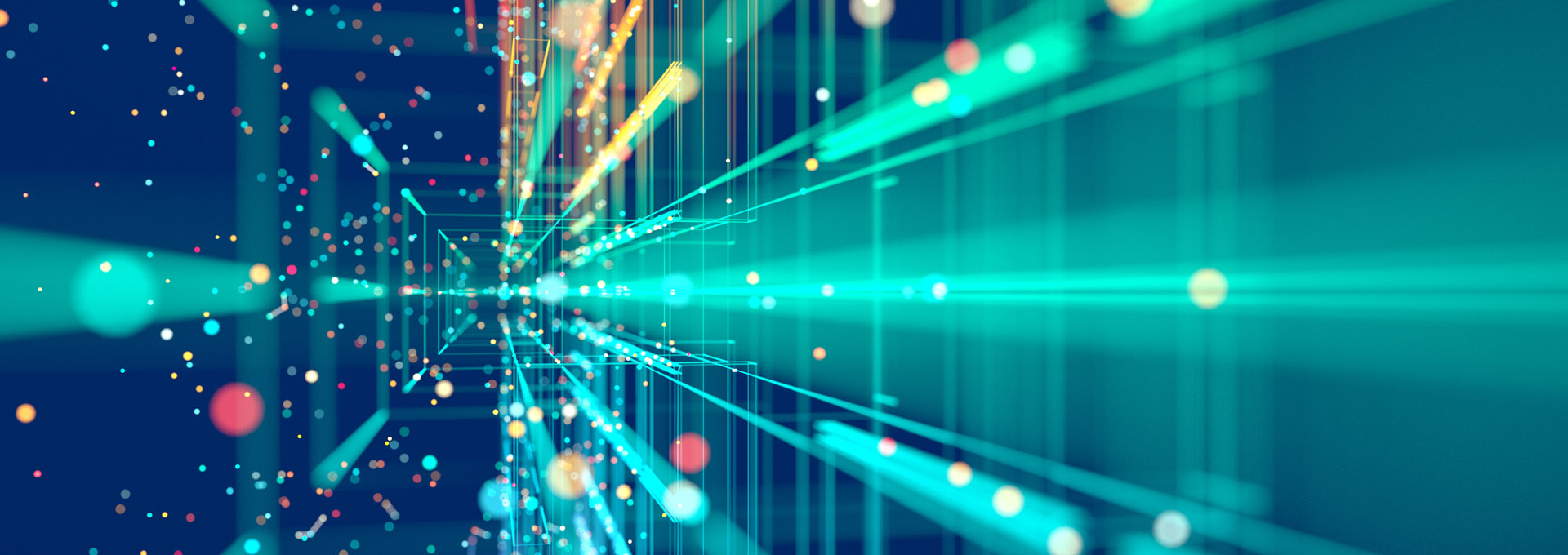
How do you get a robust manufacturing process in pharma and biotech?
A theoretical answer would consider all the involved variables and constants that determine the system to establish the dynamic state equations that characterize the process. However, the laws of physics, chemistry and engineering force us to make approximations because the available data does not always explain the entire context. Taking real-time data of all the variables that must be taken into account to apply the theoretical model is an arduous, if not impossible task, because the multitude of information sources are usually not integrated or not available.
This scenario has drastically changed with the application of AI (artificial intelligence) in industrial environments.
During the 2020 PDA Data Integrity Workshop, the role of big data and AI under the biopharma manufacturing perspective was introduced to participants, with an emphasis on the differences between AI and big data, good practices, and real-use cases with AI. A discussion of multiple AI algorithms brought to light how to improve the manufacturing process using good data, emphasizing one of the main takeaways from the workshop: quality data is the starting point for AI applications in pharma and biotech.
Actually, AI algorithms only can provide realistic AI models when quality data is used to train the models and this topic was emphasized during the workshop. In the course of the AI workshop, several poll questions were popped up to the pharma audience. The answers of the attendees made a composition of the state of AI adoption in the pharmaceutical industry.
A total of 87 attendees participated in the session, of which 51% were representatives of pharmaceutical companies, 23% were consultants, 11% were regulators, 2% were manufacturers of equipment for the pharmaceutical industry, and the remaining 5% were from the PDA organization.
Participants were asked several questions regarding their use of data analytics approaches and tools and data integrity management.
The first set of questions was related to the general data analytics approaches that are being used in their organization. In total, 84% of respondents reported that they do not use chemometric methods to analyze their data problems. In a question about how often they use XY linear regression (bivariate) analysis, almost three-quarters of participants (74%) responded that they use such methods only rarely or sometimes, whereas 26% of participants use XY linear regression usually or very often. Similarly, when asked how often they use multivariate analysis (analysis of more than two variables at once), 14% of respondents reported very frequent use, whereas 38% of respondents use such methods usually and 48% only rarely.
More insight was obtained when participants were asked about obstacles and challenges in implementing data analytics in their process.
Almost 80% of respondents reported that the most time-consuming activity in the data analysis pipeline is data collection and data cleaning, while 20% reported the most time-consuming activity is result validation. In line with this result, 80% of the respondents indicated that they have not attempted to validate or in any way evaluate their in-use AI algorithms. This highlights that the most time-consuming tasks are generally related to data availability and data quality, rather than higher-level tasks such as choosing the most appropriate algorithms or putting AI model to production.
Therefore, the still incipient deployment of AI in the pharmaceutical industry leads do not contemplate quality tasks in the governance of algorithms and models such as AI validation. Nevertheless, there are some initiatives already in place describing strategies to qualify AI algorithms based on Quality by Design principles (1) establishing the foundation for the usage of AI in GxP environments.
When new data-related problems must be tackled, 38% of the participants reported that the usual practices they apply to the data are applying standard operating procedures (SOPs) to manage the raw data and data transformations, 31% of the participants reported that they look for anomalies and random effects, and 15% of the participants reported they manage outliers in the data. Only 10% of the participants indicated that they keep data under the right governance procedure.
The participants were asked about their general opinion regarding the required quality around AI. Worryingly, two-thirds of the participants think that the Pharma, Biopharma, and medical devices industries are not ready to implement AI in their manufacturing processes. Similarly, 59% of the respondents believe that pharma, biopharma, and medical devices are not ready for AI implementation in general across the regulated industry. Finally, participants were asked about which particular hurdle their organization has experienced in the implementation of AI. The most significant obstacles reported are the lack of resources (38%), regulation (23%), and validation (21%), followed by insufficient budget (10%) or data (8%).
Overall, the poll question results indicate that there is a lot of work to be done to disseminate good data and AI implementation practices and encourage adoption of the technologies. Nevertheless, a McKinsey study suggests that there will be a significant disparity in benefit for manufacturers who are early adopters of AI verse those who hesitate on advancement and are thus left behind (2). As the graph below shows, leaders can expect a 122% increase in cash flow, compared to a 10% cumulative change seen by followers.
There is a promising horizon for the AI adoption in biopharma and at the same time, a good opportunity to improve some existing issues in regards of data integrity. On the first hand AI algorithms require good and quality data to generate valid AI models. On the other hand, the inherent complexity and continuous variability on biomanufacturing processes only can be driven by advanced systems based on expert algorithms which are able to reproduce the reality. This is an ideal combination of factors that is accelerating the AI adoption in the biopharmaceutical industry.
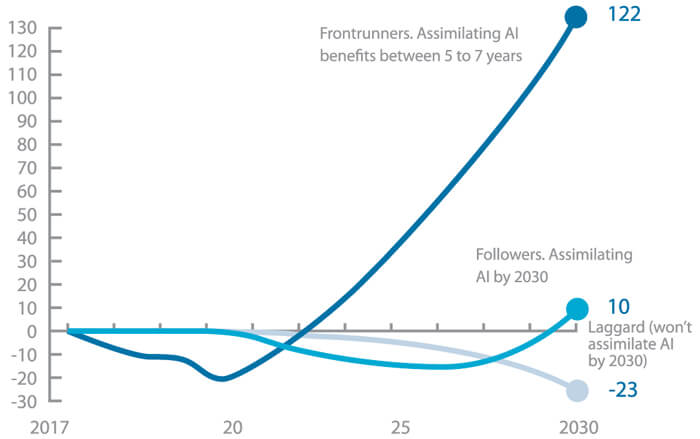
References
- Manzano, T.; Fernandez, C.; Ruiz, T.; Richard, H.; AI Algorithm Qualification; PDA JPST;
DOI: 10.5731/pdajpst.2019.011338. - McKinsey Global Institute Analysis, “Lighthouse’ manufacturers lead the way—can the rest of the world keep up?”; 2019.