How AI and ML Is Getting Integrated into Life Sciences Companies
When ChatGPT launched in November 2022, it went viral, rocketing to "dinner table brand awareness" within weeks. It shattered Instagram’s
records for the fastest app to reach one million users and then 100 million users (see Figure 1).
Why? Was it only because people were enamored with the novelty of writing a tribute to their favorite sports team in the style of a Shakespearean sonnet? No. It was at least as much due to the ease of the interface. You simply asked, and the chatbot responded. You could have something resembling a human-to-human conversation.
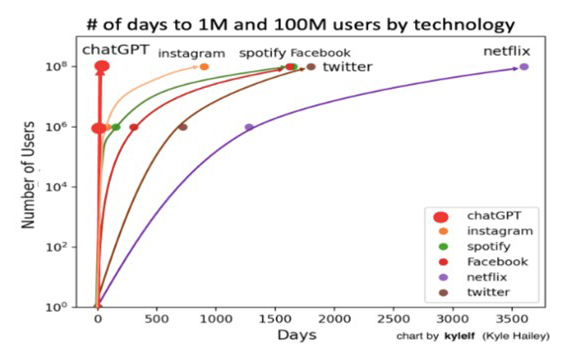
This revolution in the interface became even more evident and powerful with data analysis. Figuring out which 10 stocks in the S&P 500 offer the best dividends might have taken a while previously, but now the answer comes back in seconds. If you wanted to ask a follow-up question, like which of those ten stocks have appreciated by at least 8% over the past year, that answer also took just seconds.
Even though this ChatGPT capability seemed completely new in November of 2022, it was built upon the foundation of years of previous work by companies large and small all over the world. However, it was the combination of the chat interface and the scope of the ChatGPT knowledge base that turned ChatGPT into a household name.
As William Gibson said, “The future has already arrived. It’s just not evenly distributed yet.” ChatGPT distributed it to everyone through a better interface.
As the CEO of a data analytics firm serving life sciences customers, my first thought was how will this impact the life sciences industry and how will it impact our own product roadmap?
Before we discuss how artificial intelligence and machine learning (AI/ML) will impact the life sciences industry, let us look at the verticals it is most likely to impact the most across all industries.
-in-drug-discovery.jpg?sfvrsn=9fb8434d_1)
The most impacted verticals are likely to be sales and marketing, software engineering, customer ops, supply chain and ops, and product research and development (R&D). Within life sciences, it is mostly product R&D, sales and marketing, and software engineering. We have seen that over the past year or two with big headlines in fields like drug discovery in pharma and software-defined devices in medtech (see Figure 2).
Aspects of quality assurance, compliance and regulatory surveillance are less likely to be deeply impacted, at least in the nearish term. That is because the revenue opportunities unlocked by meaningful application of AI/ML in quality assurance do not seem quite as compelling as they are for drug discovery.
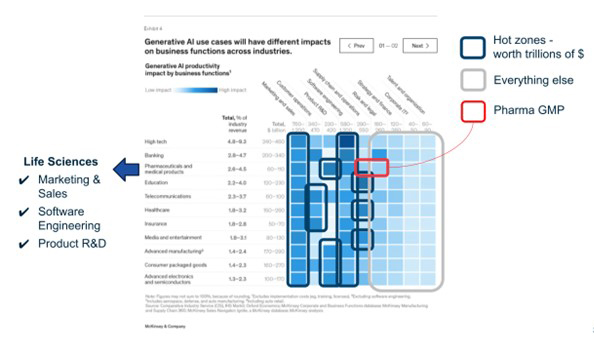
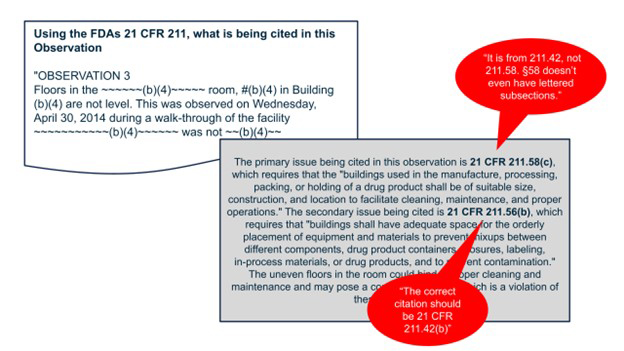
There are also still difficult data structuring and cleansing challenges. AI does famously “hallucinate.” It will confidently present incorrect information, especially when there is a need for high-domain context to produce accurate answers when the user has a low tolerance for inaccurate responses, which makes the risk of using AI not worth the effort. We tested that by asking AI agents to respond to prompts like: “Using the U.S Food and Drug FDA’s 21 Code of Federal Regulations (CFR) 211, what is being cited in this 483 Observation?” It will come back with an answer that sounds plausibly correct but may be inaccurate, like citing non-existent sections of the CFR (see Figure 3).
So, how can we successfully apply AI/ML to areas of life sciences like quality assurance and compliance? Surely, we can do better than today’s version-control nightmare of SharePoint sites filled with spreadsheets, decks, PDFs, homegrown dashboards and quality management system applications.
We are taking the following approach:
- Double down on our efforts to optimally structure unstructured data.
- AI/ML struggles to perform effectively with messy data. Common data structure challenges we address include entity resolution (e.g., why multiple facility establishment identifiers exist for the same site name), entity linking (e.g., identifying the parent company of a site using our Knowledge Graph) and extracting relevant data from text within PDFs (see Figure 4).
- Build guardrails around our AI/ML to eliminate hallucinations.
- Techniques like Retrieval Augmented Generation helps reduce large language model hallucinations by setting a boundary of knowledge it can apply to the answers.
- Ensure that the supplied answer is relevant to the specific task at hand.
- Building task-specific AI agents that are trained on specific data sets to execute well-known workflows towards a desired outcome.
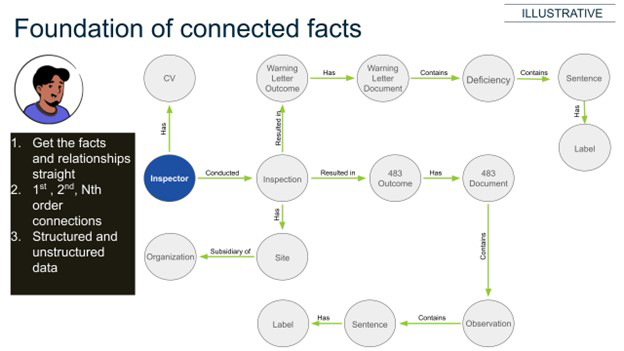
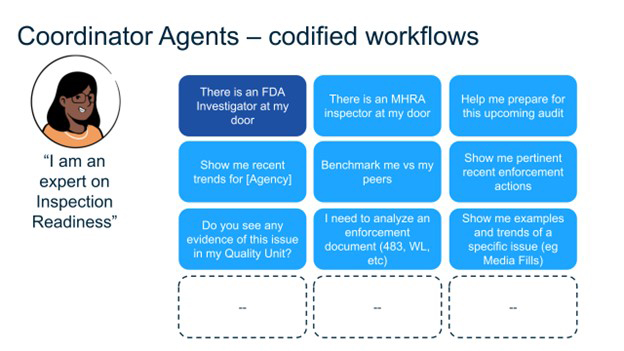
What does the future hold? One thing is certain: things will evolve quickly and in unpredictable ways. Like most technology, today’s version will be the worst you will use. Here are some of my predictions:
- The chat interface will become the dominant interface for software applications.
- The dashboards we know (and love?) of today will fade into the background.
- We know more about what is in our breakfast cereal than we do our medications…that will change despite the secrecy of the life sciences industry.
